New project will focus on predicting daily PTSD and cannabis use disorder symptoms among veterans
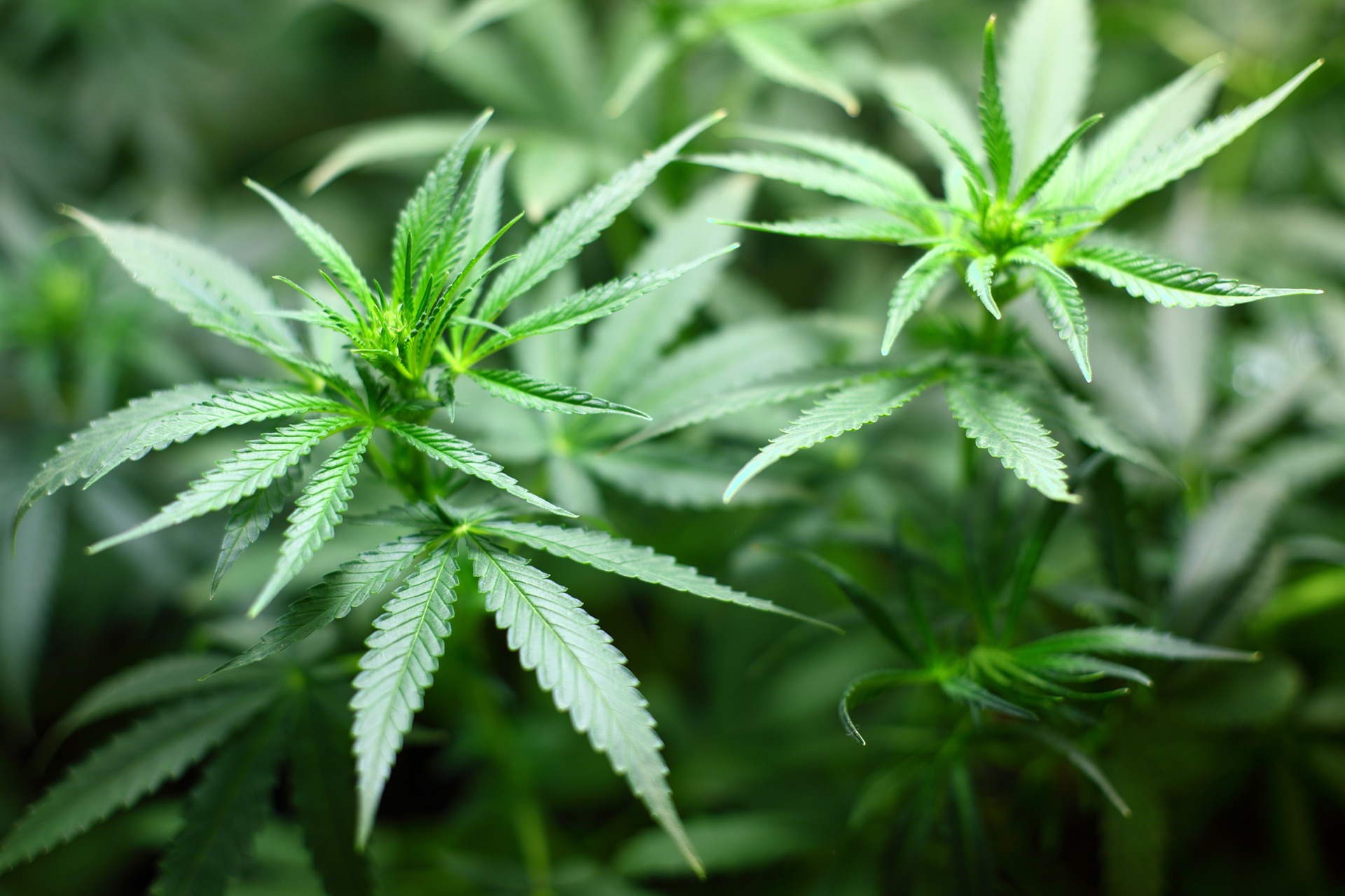
This study will investigate veterans’ use of cannabis in relation to PTSD symptoms.
USC CAIS Associate Director Jordan Davis and USC CAIS Co-Director Bistra Dilkina recently received a grant from the National Institutes of Health to begin a study that uses machine learning to predict daily PTSD and cannabis use disorder symptoms among non-treatment seeking veterans.
Davis and Dilkina will apply ML algorithms to determine whether passive data from smartphones and wearable devices are useful to better understand the interplay between the two conditions. “There have been some great advances in ‘just in time’ interventions, however we want to develop algorithms that use machine learning that can predict when someone will experience escalations in symptomatology that reach a clinical threshold. Our goal is to utilize as much data as possible including user input, passive data, as well as data that wearable technology puts out. Doing this may give us a better sense of which factors predict escalations and how much heterogeneity exists in these data across risk profiles,” said Davis. While the field is still emerging, prior work shows PTSD diagnosis was predicted with 82% accuracy by applying ML algorithms to trauma narratives alone 44 and with 94% accuracy using 4 seconds of voice data.
The study will also combine active data collection to determine whether passive data alone is enough or if both are necessary to best predict symptom escalation during a critical period. Understanding the feasibility and acceptability of passive data collection in clinical practice among veterans is also an important part of the study.
Davis believes that the most challenging part of their research will be data standardization and data quality: “We have so much data that is transmitted every minute, every day, yet not much of it is standardized across devices. Our job will be to aggregate this data and find a way to make it easily accessible to treatment providers and patients. While this is only the first phase of this line of research, the end goal would be to have algorithms that can deliver just in time interventions as an adjunct to regular treatment. That requires data synchronization as well as aggregation in a digestible way. This raises more questions than answers, but that’s why we do it!”
The project, which started September 1st, features an interdisciplinary team composed of experts in the areas of PTSD/CUD comorbidity (Davis, Eric Pedersen, Emily Dworkin), veteran populations (Pedersen, Daniel Leightley), daily diary methods (Dworkin), passive data collection (Leightley), and ML models (Leightley, Dilkina). Eric Pedersen, PhD, is an associate professor of psychiatry and the behavioral sciences at the Keck School of Medicine of USC. Emily Dworkin, PhD, is a licensed clinical psychologist and an Assistant Professor in the Department of Psychiatry and Behavioral Sciences at the University of Washington School of Medicine. Daniel Leightley, PhD, is a postdoctoral research associate at The King’s College in London.
About Jordan Davis: Jordan Davis is an assistant professor in the department of children, youth and families at the USC Suzanne Dworak-Peck School of Social Work. Davis is also an Associate Director of the USC Center for AI in Society (CAIS), a joint effort between the USC Viterbi School of Engineering and the USC Suzanne Dworak-Peck School of Social Work. He has devoted much of his career to research addressing substance use and the developmental needs of marginalized and vulnerable populations. Davis also focuses on the utility and development of longitudinal data analyses in the structural equation modeling framework. Davis’ primary research focuses on substance use disorder treatment among marginalized youth. His intervention work mainly concentrates on mindfulness-based relapse prevention and how it can be utilized to address issues facing at-risk youth.
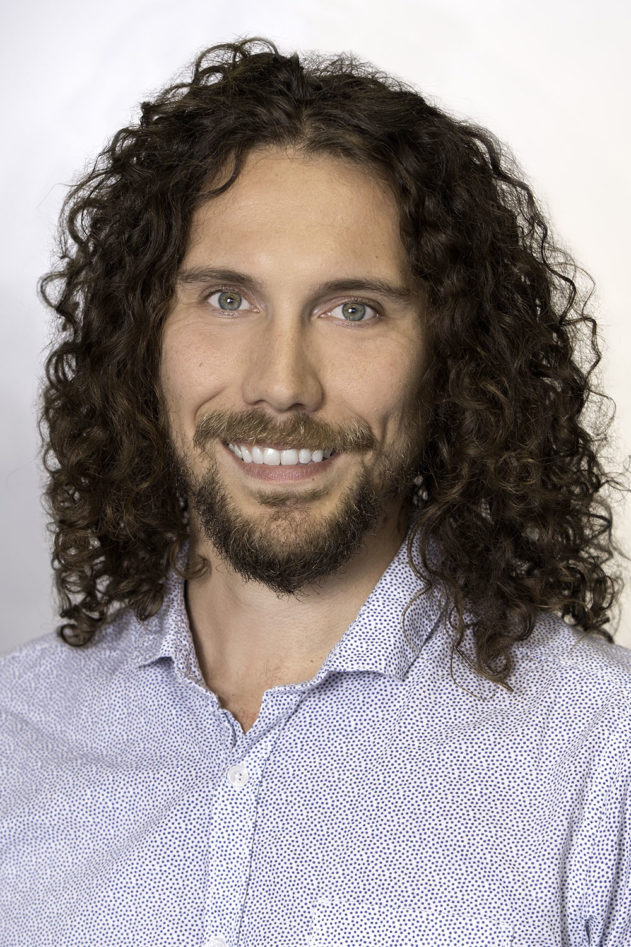
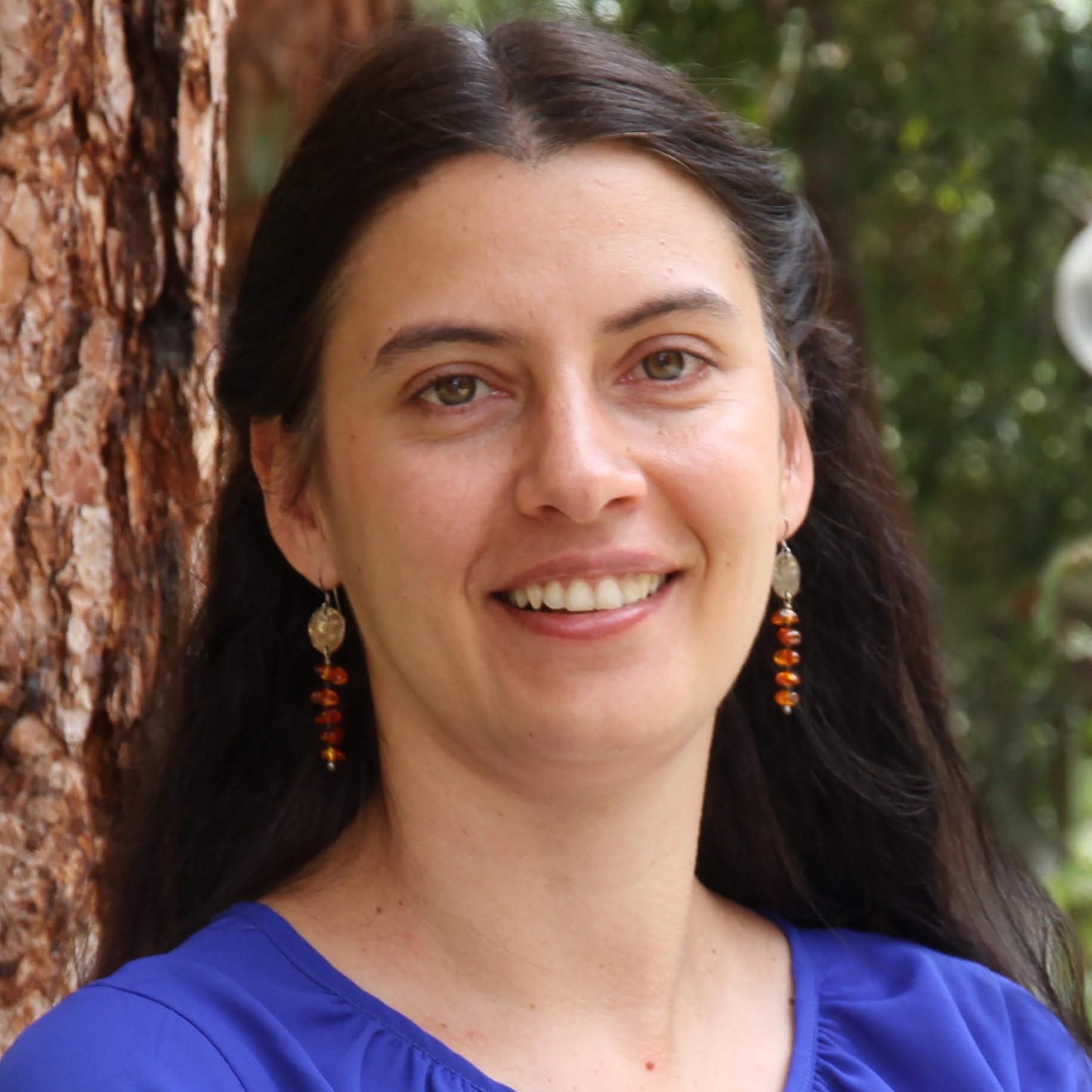
About Bistra Dilkina: Bistra Dilkina is an associate professor of computer science at the USC Viterbi School of Engineering. She is also a Co-Director of USC CAIS. Dilkina’s research focuses on advancing the state of the art in combinatorial optimization techniques for solving real-world large-scale problems, particularly ones that arise in sustainability areas such as biodiversity conservation planning and urban planning. Her work is at the intersection of discrete optimization and machine learning. One key area of research is designing machine-learning-driven combinatorial optimization algorithms, by leveraging the plethora of data generated by solving distributions of real world optimization problems.