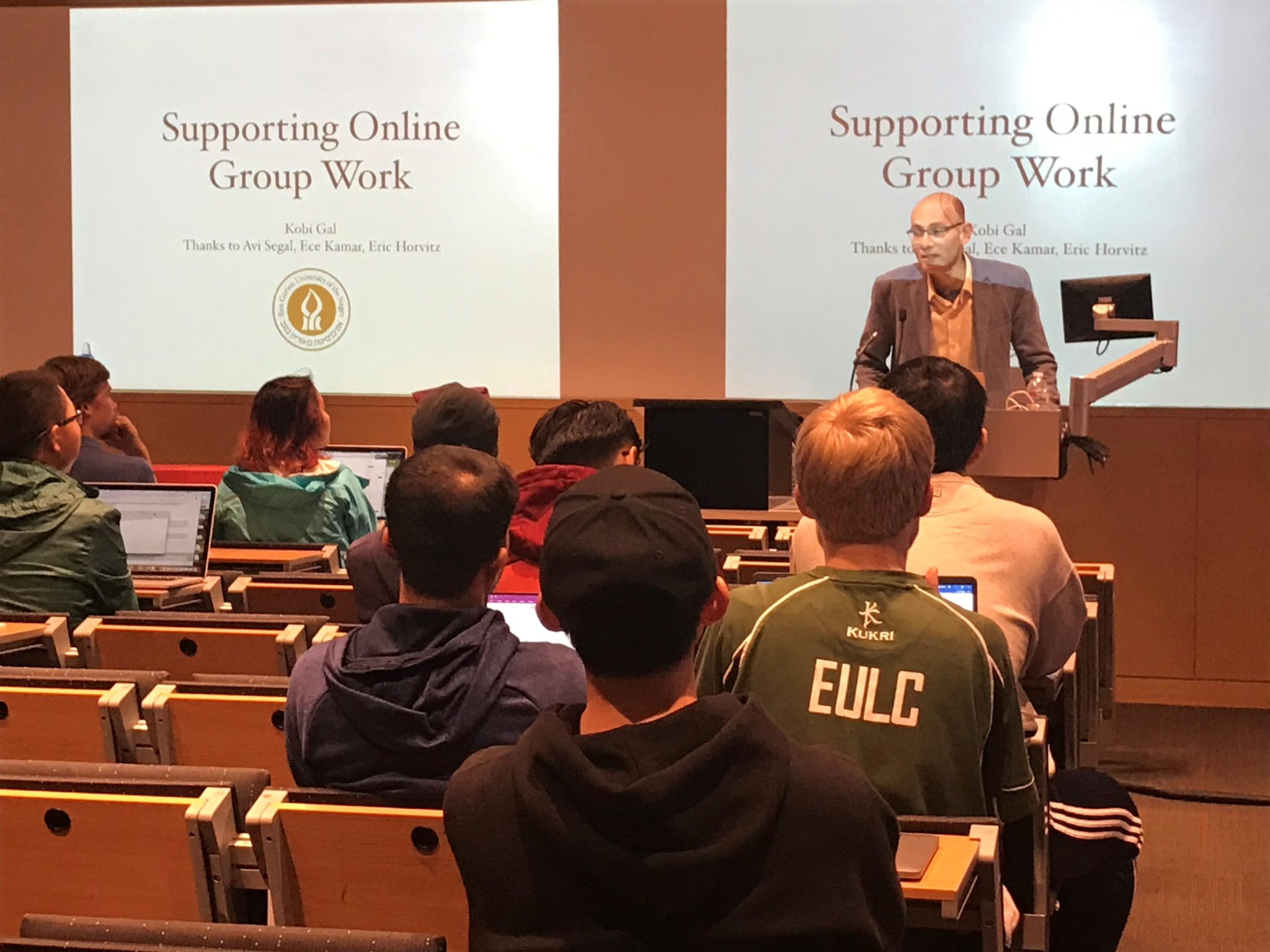
Have you heard of eBird, Globe at Night, or Old Weather? Perhaps you’ve even used one of these platforms to report bird observations, record the number of stars you see at night, or transcribe old logbooks with weather observations. These are all examples of citizen science, a growing field in which the public participates in scientific research by providing data collection, analysis, or reporting. Every action by participants helps to advance scientific efforts, so keeping these volunteers engaged is critical. Dr. Kobi Gal from the Ben-Gurion University of the Negev has been working to support citizen science by using intelligent intervention to encourage continued participation.
Galaxy Zoo is an astronomy project that enlists the public to help classify large numbers of galaxies. The project has had over one million users assist with classifying hundreds of thousands of galaxies. There are a number of “superusers” who have volunteered countless hours and contribute to the site’s active discussion forum. The majority of users, though, click through a handful of galaxies and never return—80% of first-time visitors never log back on.
To motivate continued participation in the largest galaxy census ever, Dr. Gal has looked into ways to incentivize people to interact with these citizen science efforts for longer. In a paper published in AAAI18, his group introduced an approach for “intelligent intervention.” They used reinforcement learning to develop an optimal policy for displaying motivational messages to encourage user engagement.
Dr. Gal described many factors that impact the effectiveness of such an intervention: population, delivery, content, timing, and duration. His group used random intervention trials to determine the most effective policy based on these factors. Considering content, one message told users, “You’re doing a wonderful job!” Another message promoted being part of the Galaxy Zoo community; the third variant attempted to alleviate the anxiety users may feel about providing inaccurate classifications. They found the first message, reminding users how helpful they were in this scientific effort, most successful. Another important factor was the timing of these motivational messages. They found that randomly-timed messages actually hurt the user’s productivity when compared to a control group that received no intervention. However, when they used machine-learning algorithms to predict when users were likely to leave, the messages successfully motivated users to classify significantly more galaxies.
Using an optimal policy based on all of these factors, Dr. Gal’s intervention efforts significantly increased engagement among thousands of users, directly benefiting one of the largest citizen science projects. His work adds to the growing body of literature that demonstrates ways to leverage AI for intelligent intervention towards positive causes.