Big Data in Climate: Opportunities and Challenges for Machine Learning and Data Mining
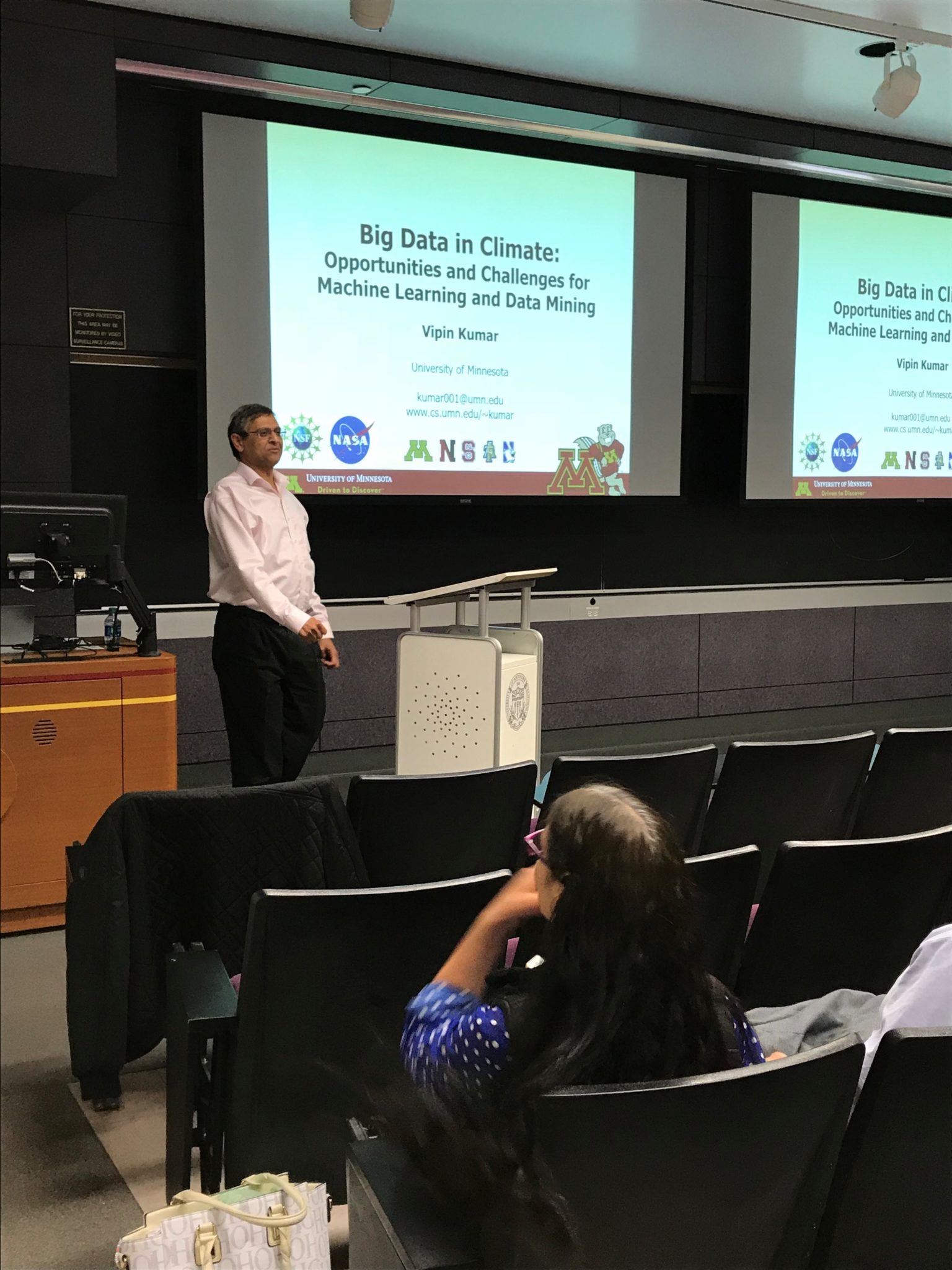
Dr. Vipin Kumar presenting at a USC CAIS seminar.
In the past, there were very limited data about climate. The past 30 years has seen an explosion of climate data from satellites. This creates the unique opportunity for computer scientists to use computational techniques to analyze such big data. Despite the rich literature of the data mining field, many new challenges arising in this particular domain (e.g., unique data structure, lack of labeled data, the underlying physical rules, huge amount of data heterogeneity) requiring fundamental new techniques.
This talk concerned the problem of monitoring global challenges, and illustrated this theme with three case studies: 1. Global mapping of forest fires, 2. Mapping of plantation dynamics in tropical forests, 3. Global mapping of inland surface water dynamics.
Due to limited time, the talk mainly focused on the first point – model the global mapping of forest fires. There are several major challenges in this problem: imperfect labels for supervision, highly imbalanced classes, and how to evaluate performance of a model using imperfect labels. Dr. Kumar spoke about the approach to handle the first challenge. By exploring the structure of the data as well as physical principles underlying the problem, they were able to develop machine learning models that perform almost as well as the case with perfectly labeled data.
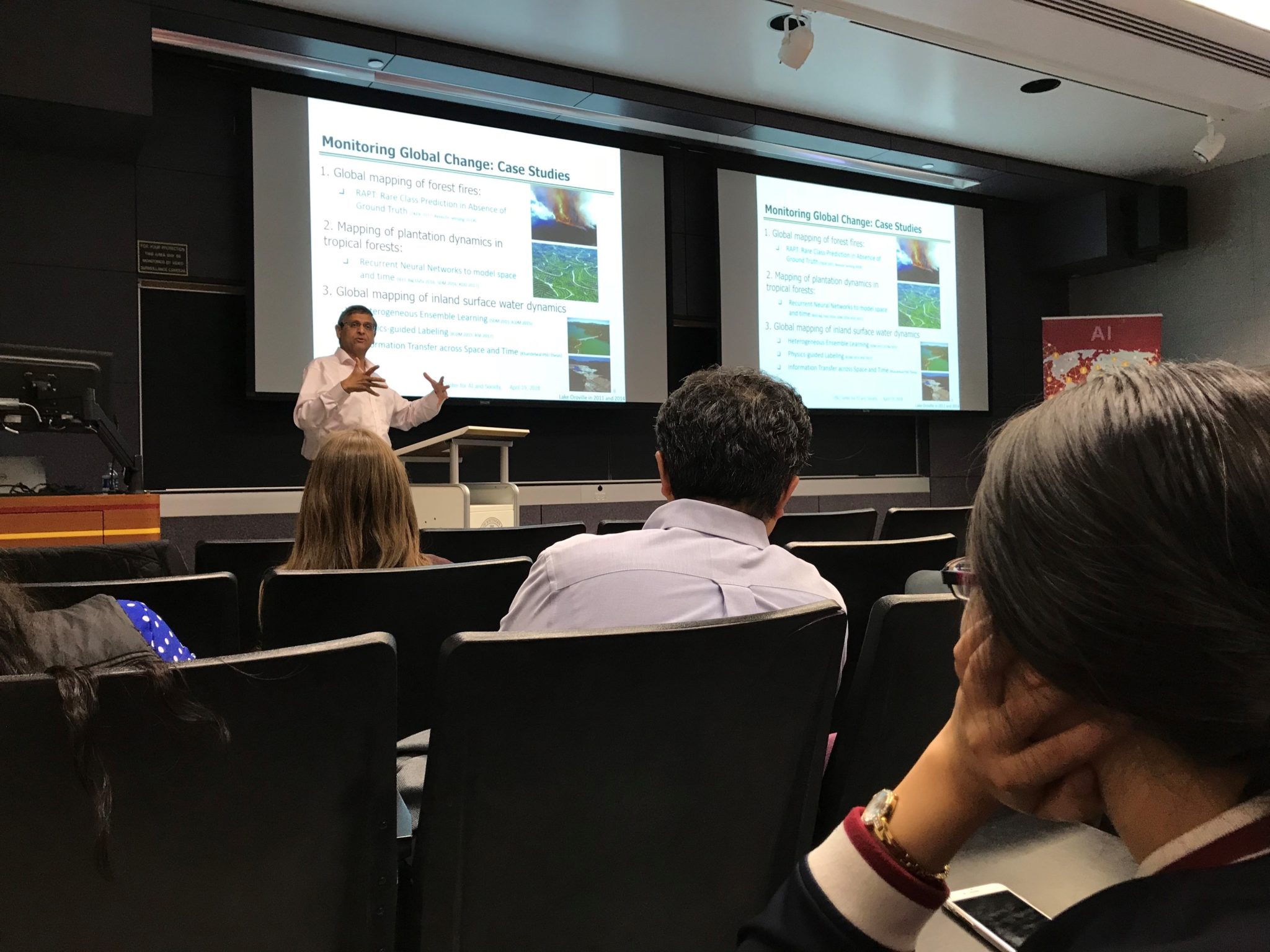
A very important outcome of this research is that they can increase our understanding about the maps of fires, forest, etc. This provides very valuable information to assist local agencies’ decision making. For example, by predicting the resulted fire area due to the burning of a plantation, this can help the rangers know which areas would be more important to patrol and which may be less important.