USC CAIS Associate Director Phebe Vayanos is developing AI to help policymakers allocate limited housing resources more fairly and efficiently.
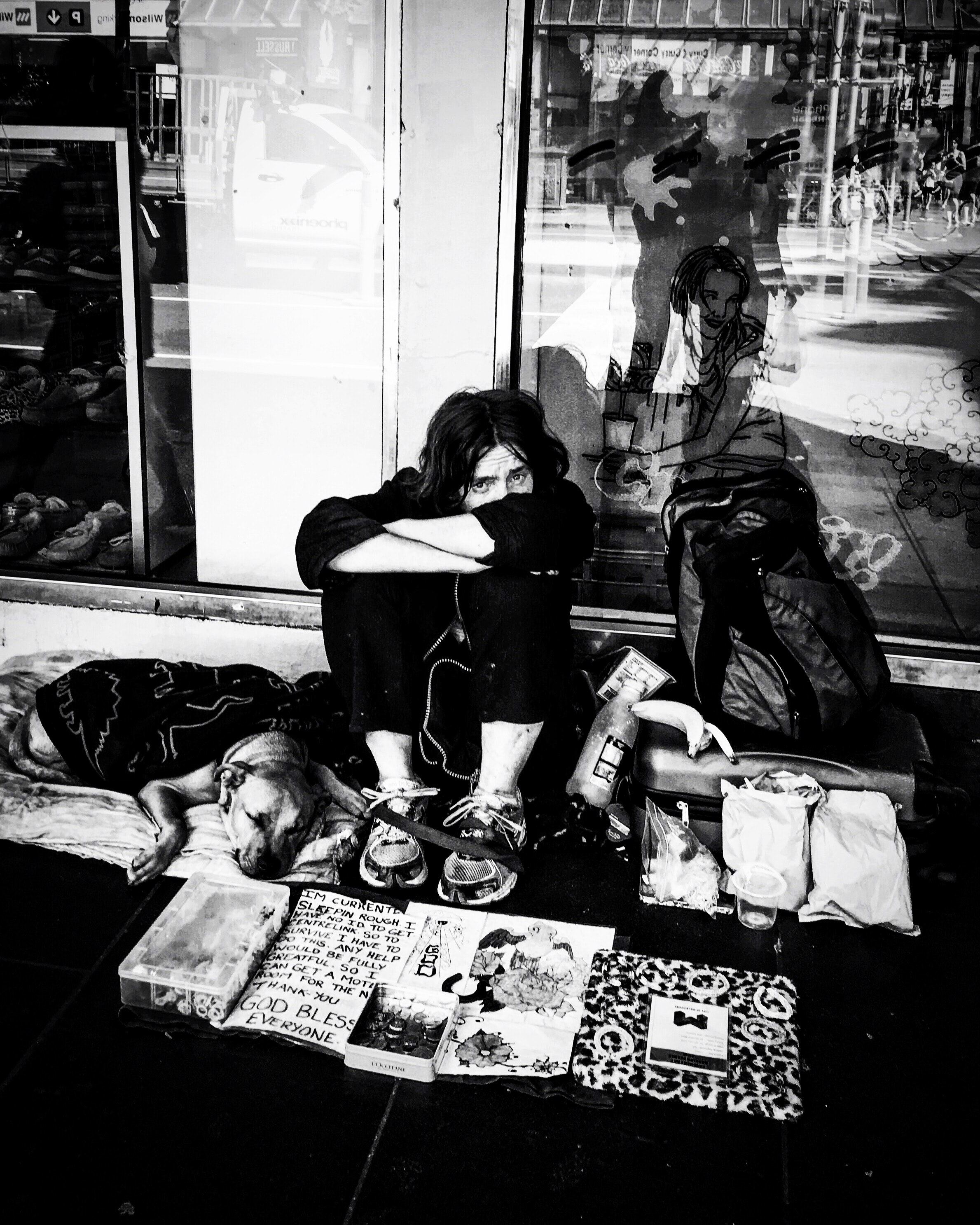
The rate of homelessness is climbing in Los Angeles, up 12 percent in the county and 16 percent in the city from 2018. With more than 36,000 homeless residents to serve, the city’s authorities struggle to find new housing options and optimize existing resource allocation.
Research consistently indicates that people of color are at increased risk of experiencing homelessness. Unfortunately, even after accessing housing assistance services, many members of ethnic and racial minority groups face disproportionate disadvantages to securing stable housing. In fact, a recent report from the Los Angeles Homeless Services Authority (LAHSA) reveals that 14.2 percent of African Americans would be homeless again within a year after enrolling in permanent supportive housing programs—nearly double the rate of their White counterparts and significantly higher than that of Latinos (8 percent).
This begs the question: what can policymakers and social services agencies do to ensure their resources are being distributed not only more efficiently, but also more fairly?
Phebe Vayanos, assistant professor at the USC Viterbi School of Engineering and Eric Rice, associate professor at the USC Suzanne Dworak-Peck School of Social Work, teamed up to answer this question at the USC Center for Artificial Intelligence in Society (CAIS), where Vayanos serves as an associate director and Rice is a co-founder and director. Together, they are developing algorithms able to identify levels of need in order to create greater equity in housing resource allocation.
The problem of limited resources
Longer-term community housing resources typically fall into two categories: permanent supportive housing (the most comprehensive option) and rapid rehousing (a shorter-term program that provides rental assistance to help individuals and families get back on their feet). Due to the sheer number of homeless individuals and families in LA, services must be prioritized.
“Resources are very limited,” Vayanos said. “Policymakers are given the immense responsibility of deciding who gets access to stable housing and who does not.”
Policymakers and social workers currently rely on a homeless vulnerability score, calculated using a number of key factors, including age, health and more, to match individuals to the appropriate public housing resources. According to this system, those whose score deems them the most vulnerable are offered permanent supportive housing, those who are least vulnerable are not eligible for public housing and those whose risk level falls somewhere in between may be offered rapid rehousing.
While seemingly straightforward, a potential limitation of this policy, according to Vayanos, is that it is not tied to outcomes. “We conducted a study evaluating the efficiency and fairness of housing interventions for approximately 11,000 homeless youth and found that the current policy actually proved less efficient than random allocation—meaning it did not lead to more individuals exiting homelessness,” Vayanos said. “It also resulted in unfair outcomes for Black and Latino youth.”
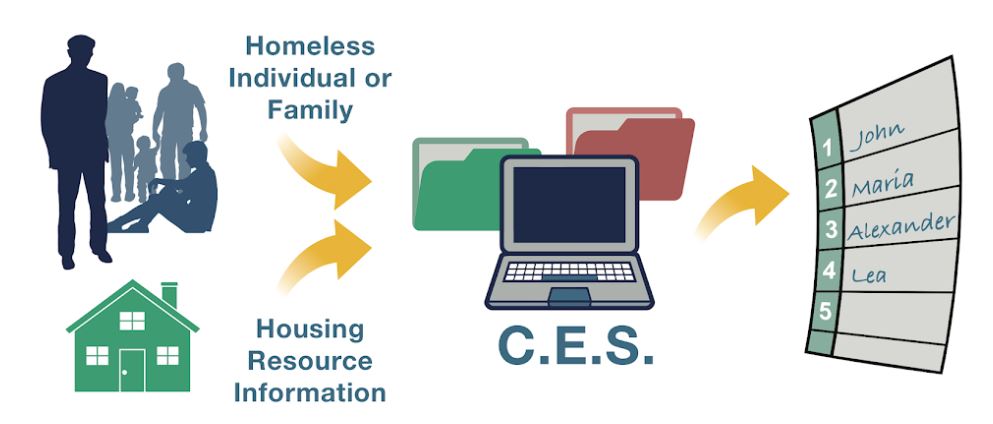
Leveraging AI to inform policymaking
To help policymakers better prioritize and distribute their limited housing resources, Vayanos and Rice have developed new AI algorithms (and here) and theoretical frameworks that can be customized to reflect the unique goals of each community.
“We know that no two communities, and no two policies are the same, and we wanted to produce a theoretical model that reflects that,” Vayanos said. “We conduct analytical assessments by which policymakers can specify their top outcome goals—essentially measuring the respective importance of efficiency, fairness and interpretability.” The results from this preference elicitation step enable Vayanos and her team to then design a housing allocation policy (a scoring rule) whose outcomes are tailored to meet the policymaker’s priorities. The scoring rules can be based on a comprehensive evaluation of individual characteristics including age, substance use history and geographic location.
Once the framework is in place, and each time a housing resource is procured, a score is assigned to each individual still on the waitlist and the housing resource is assigned to the person with the highest score.
While the algorithm has yet to be deployed, the team aims to run a pilot of the preference elicitation in the fall 2019 and a pilot of the associated policy in the summer 2020. Initial findings have proven promising. “In a test case on an administrative data set of youth experiencing homelessness across 16 communities in the US, we saw 16 percent more youth successfully transition out of homelessness and we documented a reduction in racial disparities in outcomes by a staggering 72 percent,” Vayanos said.
The future of AI in eradicating homelessness
Vayanos emphasizes that while there is no easy solution to homelessness, AI has the potential to effect real change.
“There are so many variables when it comes to alleviating homelessness and we’ve only scratched the surface,” Vayanos said.
At the heart of Vayanos’ efforts is a desire to make an impact within her community and aid those in need. “I want to leverage my passion for both computer science and social justice to help provide a sense of hope and stability to those experiencing homelessness,” she said.