Housing & Homelessness-Related Projects
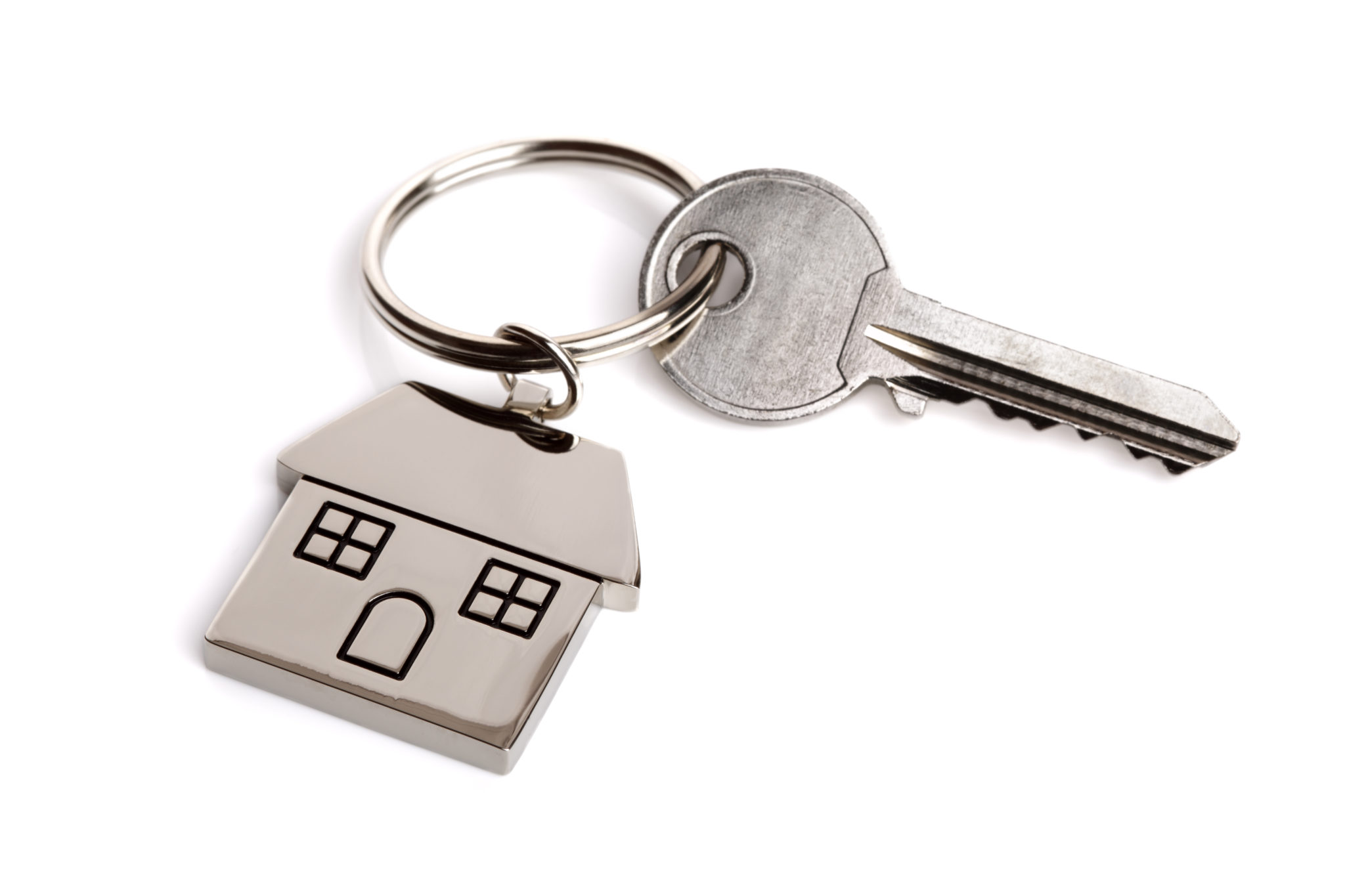
Understanding user experience with a housing resource allocation decision aid for youth experiencing homelessness
Naveena Karusala & Jennifer Wilson
The TAY-VI-SPDAT: Next Step Tool (NST) was developed for use as a pre-screening tool for homeless youth aged 24 and younger to assist in prioritization and allocation of housing resources. Currently, the NST is being used by agencies and services providers in over 35 states across the county. While research has been done to evaluate the effectiveness of NST scores in determining housing need and type among young people experiencing homelessness, little is known about the user experience of community-level policymakers and service providers with the tool. Furthermore, work being done by USC CAIS summer fellows to apply machine learning to the NST has the potential to improve upon the tool by providing a more nuanced housing prioritization and allocation score. (See the project titled, “Developing efficient, fair, and interpretable housing prioritization tool to address youth homelessness” for more information.) However, input from the end user is required to inform and guide this process. Thus, through qualitative interviews and focus groups with Los Angeles-based policymakers and services providers currently using the NST, as well as youth experiencing homelessness who may be impacted by such policies, this research seeks to understand the experience of using the NST as a housing resource allocation decision aid and perceptions of applying additional machine learning elements to this process.
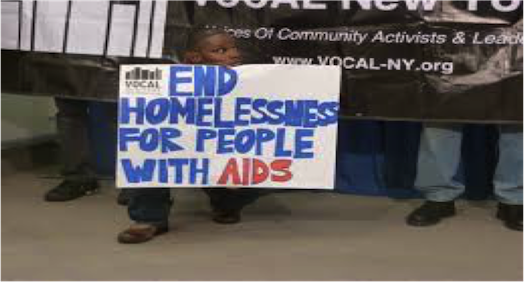
HIV Prevention among Homeless Youth: Influence Maximization and Network Diffusion for Social Good
Lindsay Young & Jerome Mayaud
This project explores the intersection of two critical crises in Los Angeles County — youth homelessness and HIV. With knowledge that homeless youth are at much greater risk of contracting HIV than stably housed populations, we aim to use Artificial Intelligence (AI) methods to heighten HIV prevention awareness and engagement in this vulnerable population. We approach this challenge from both an Influence Maximization and Network Diffusion perspective. In previous work, we developed decision support systems to help shelters and drop-in centers identify the most influential homeless youth (i.e., peer advocates) to raise awareness about HIV prevention among their peers. Now we focus on understanding how HIV-related information actually diffuses through the natural, multiplex networks of homeless youth using classic and newly developed models of network diffusion. Taken together, this work is motivated out of the desire to develop pragmatic tools and insights to help the social service community drive homeless youth toward safer behaviors.
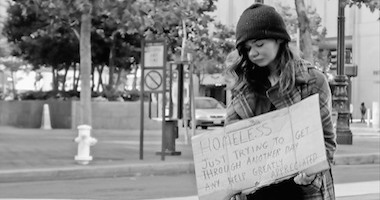
Developing efficient, fair, and interpretable housing prioritization tool to address youth homelessness
Hsun-Ta Hsu & Duncan McElfresh
The overarching goal of our project is to develop a housing allocation tool that is efficient, fair, and interpretable to community housing providers when prioritizing valuable housing resources to homeless youth. To attain the project goal, we focus our interdisciplinary efforts (e.g., computer science and social work) on the following areas: 1) evaluate current housing allocation tools on outcomes including housing stability and waiting periods; 2) gain knowledge from critical stakeholders (e.g., policy makers, housing providers, and homeless youth leaders) regarding their perceptions on efficiency, fairness, and interpretability of a housing allocation tool (see “Understanding user experience with a housing resource allocation decision aid for youth experiencing homelessness”); and 3) develop an efficient and fair housing allocation tool that is easy for stakeholders to adopt when making housing prioritization decisions.
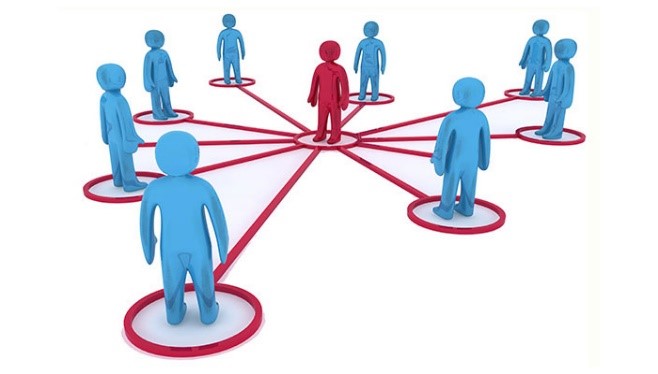
Using AI to Examine the Relationship Between Connectedness Among Runaway and Homeless Youth and Suicidal Phenomena
Jennifer Wilson & Avi Segal
Runaway and homeless youth (RHY) are at an increased risk of suicidal behavior compared to youth in the general population. An established body of literature has considered the relationship between social connectedness and suicidal phenomena, but much less is known about this relationship among high-risk runaway and homeless youth. Recent work in the RHY population found that certain features of connectedness, such as location in peer social networks, are linked with suicidal ideation. However, the nature of this relationship over time is essentially unknown, which mirrors the overall dearth of longitudinal suicide research among RHY. Overreliance on cross-sectional work makes it difficult to truly understand the predictive value of being socially connected on later suicide risk. The current research seeks to move beyond traditional statistical approaches, such as simultaneous entry regression analyses, and consider whether AI predictive models applied to longitudinal social network data can be used to provide a more nuanced prediction of suicidal behavior among high-risk runaway and homeless youth.
Conservation-Related Projects
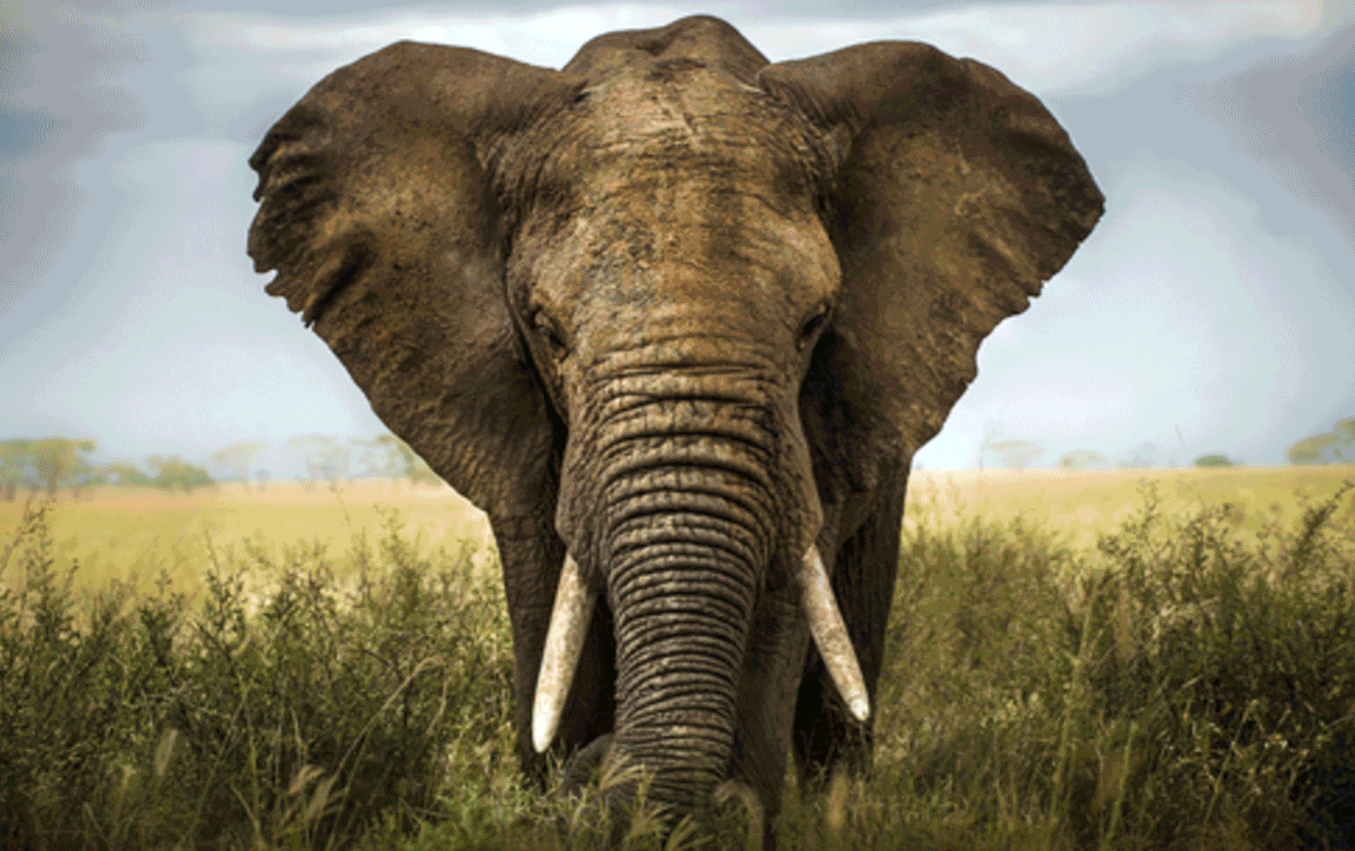
Optimization for Landscape Connectivity Conservation using Circuit Theory
Yexiang Xue
Maintaining landscape connectivity is increasingly important in wildlife conservation, especially for species experiencing the effects of habitat loss and fragmentation. We consider the problem of landscape connectivity optimization, which is to find the optimal conservation strategy of improving the resistance of a chosen subset of parcels under a budget limit in order to maximize some connectivity measure of the habitat network. Previous approaches use the length of the shortest paths as the connectivity measure, which is somewhat unrealistic in several bio-conservation settings. In this research, we assume that animals follow a random walk. We use the commuting time, i.e., the expected time for an animal to commute between two given locations, as the connectivity measure. Taking advantages of connection between random walks and the electric circuit theory, we develop novel Mixed Integer Programming (MIP) and local search solution strategies to tackle this problem.
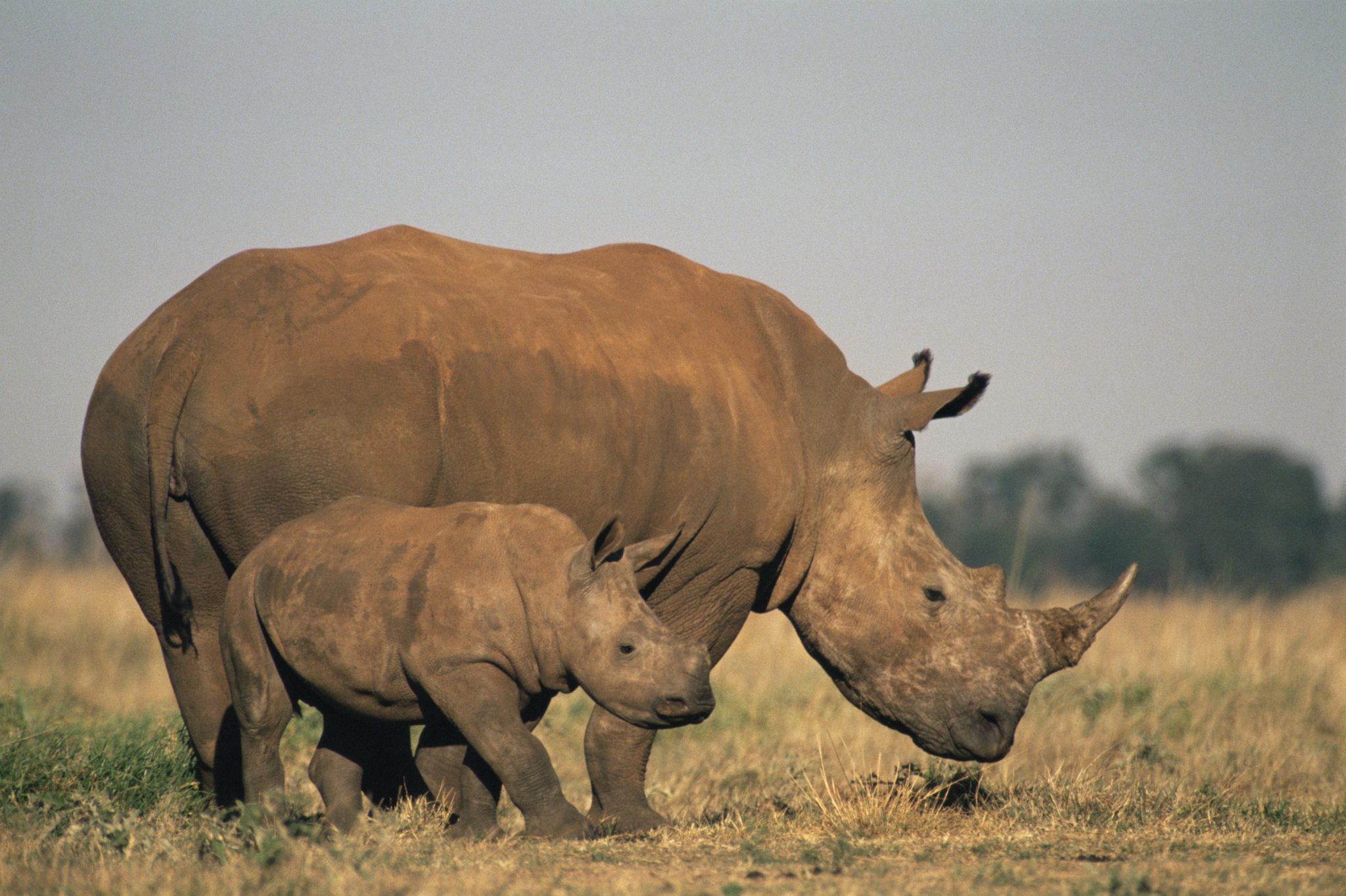
SPOT: Augmenting conservation drones for automatic real-time detection of poachers and animals
Saket Anand, Shivam Goel, & Liz Bondi
SPOT (Systematic POacher deTector) is an application that augments conservation drones with the ability to detect poachers and animals in a streaming video captured via a Thermal IR camera. The current system relies on a deep neural network architecture, Faster-RCNN, to detect and classify objects of interest in each frame independently. In its extension, SPOT 2.0, we strive toward more robust detection of poachers and animals by leveraging the temporal information in video frames. We also plan to address the data imbalance in our existing training set by augmenting it with simulated data generated from AirSim-W. Using this training data of real and simulated images, we plan to use semi-supervised domain adaptation to learn a video-based detector that achieves a higher precision and recall, taking us a step closer to full automation.
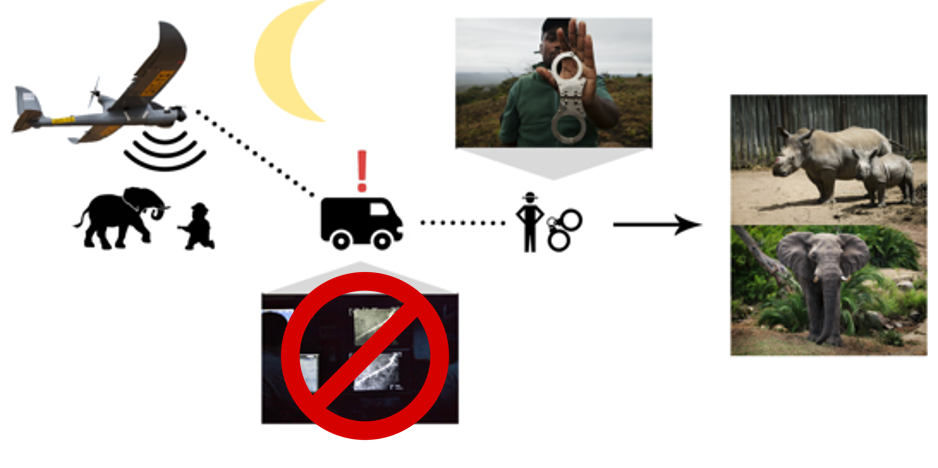
Protection Assistant for Wildlife Security (Predictive Modeling and Deployment)
Sadegh Farhang & Jerome Mayaud
In recent years, we are witnessing a decline in wildlife due to the increase in poaching activities. In this project, our aim is to tackle this issue by using machine learning. In the first phase (predictive modeling), we propose spatiotemporal machine learning models for prediction of wildlife poaching activities in different national parks in Africa and Asia. In the second phase (deployment), we provide high-risk areas based on our predictive model, which can be used by park rangers to aid them to downsize poaching activities by removing more snares and traps.
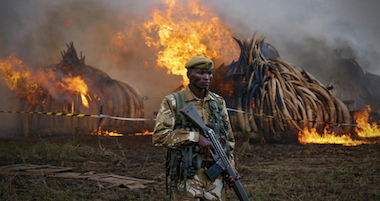
Sensor-Empowered Security Game with Uncertainty and Scheduling
Hoon Oh
In our project, we are studying sensor-empowered security game (SEG). In SEG, we have ground resources (human patrollers) and aerial resources (mobile sensors) to detect poaching activities. We are hoping to develop algorithms to compute an optimal allocation of resources and an optimal coordination strategy between human patrollers and mobile sensors. We are extending previous works in more realistic setting such as uncertainty and scheduling.
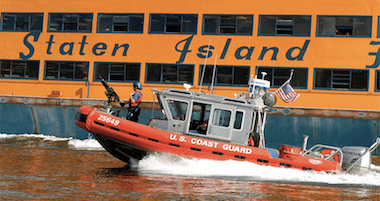
Extensive-form Stackelberg Security Game for Better Implementations in Public Safety
Xinrun Wang
When we use Stackelberg security games in normal form to plan patrols, we get probabilities over pure strategies and samples are drawn without memory of what sample was drawn previously. This means that patrols may end up not visiting some areas for a very long period of time, which is problematic for the security office when deploying the system in the real world. To address these concerns, we build the extensive-form Stackelberg security game for a more practical implementation in the real world.
Other Projects for Social Good
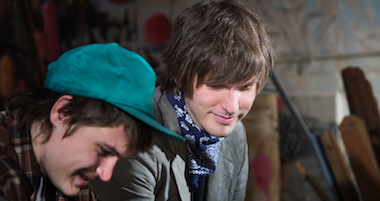
The Effect of Neighborhood Violence and Disadvantage on Stress Reactivity among Urban Youth
Sadiq Patel
Considerable effort has been devoted to understanding the behavioral and health consequences of growing up in violent and economically disadvantaged neighborhoods. These studies have focused on the relation between neighborhood crime, poverty, and youth involvement in problem behaviors–including delinquency, substance use, and aggression. Still, little is known about the physiological consequences of residing in highly disadvantaged urban neighborhoods. To address this gap in the literature, the present study examines the impact of neighborhood disadvantage and crime on stress reactivity, utilizing a longitudinal sample of 454 urban youth living in high-risk neighborhoods in Los Angeles. Furthermore, this study integrates machine learning estimation techniques to more accurately model this relation.
Eric Rice
Milind Tambe
Jordan P. Davis
Bistra Dilkina
Phebe Vayanos
Saket Anand
Hsun-Ta Hsu
Jerome Mayaud
Lindsay Young
Shivam Goel
Sadegh Farhang
Naveena Karusala
Duncan McElfresh
Hoon Oh
Sadiq Patel
Avi Segal
Xinrun Wang
Jennifer Wilson
Yexiang Xue
Liz Bondi
Bryan Wilder
Microsoft AI for Earth
Schmidt Futures