Using ML to predict daily PTSD and cannabis use disorder symptoms among non-treatment seeking veterans
Cannabis use is prevalent among Operation Enduring Freedom and Operation Iraqi Freedom veterans and rates of cannabis use disorder (CUD) have doubled in recent years. PTSD is common among veterans and in particular for those with problematic cannabis use. Co-occurrence of problematic cannabis use and PTSD is associated with greater PTSD symptom severity, decreased likelihood of cannabis cessation, worse PTSD and cannabis use clinical outcomes.
Veterans who are reintegrating to civilian life are at heightened risk of co-occurring PTSD and cannabis use. As the transition from active deployment back to civilian life (i.e., reintegration) is a difficult time (i.e., adjusting to a civilian role), it is a high-risk period for the onset or worsening of PTSD and problematic cannabis use. Unfortunately few veterans (less than 40%) who are in need of behavioral health care actually receive it.
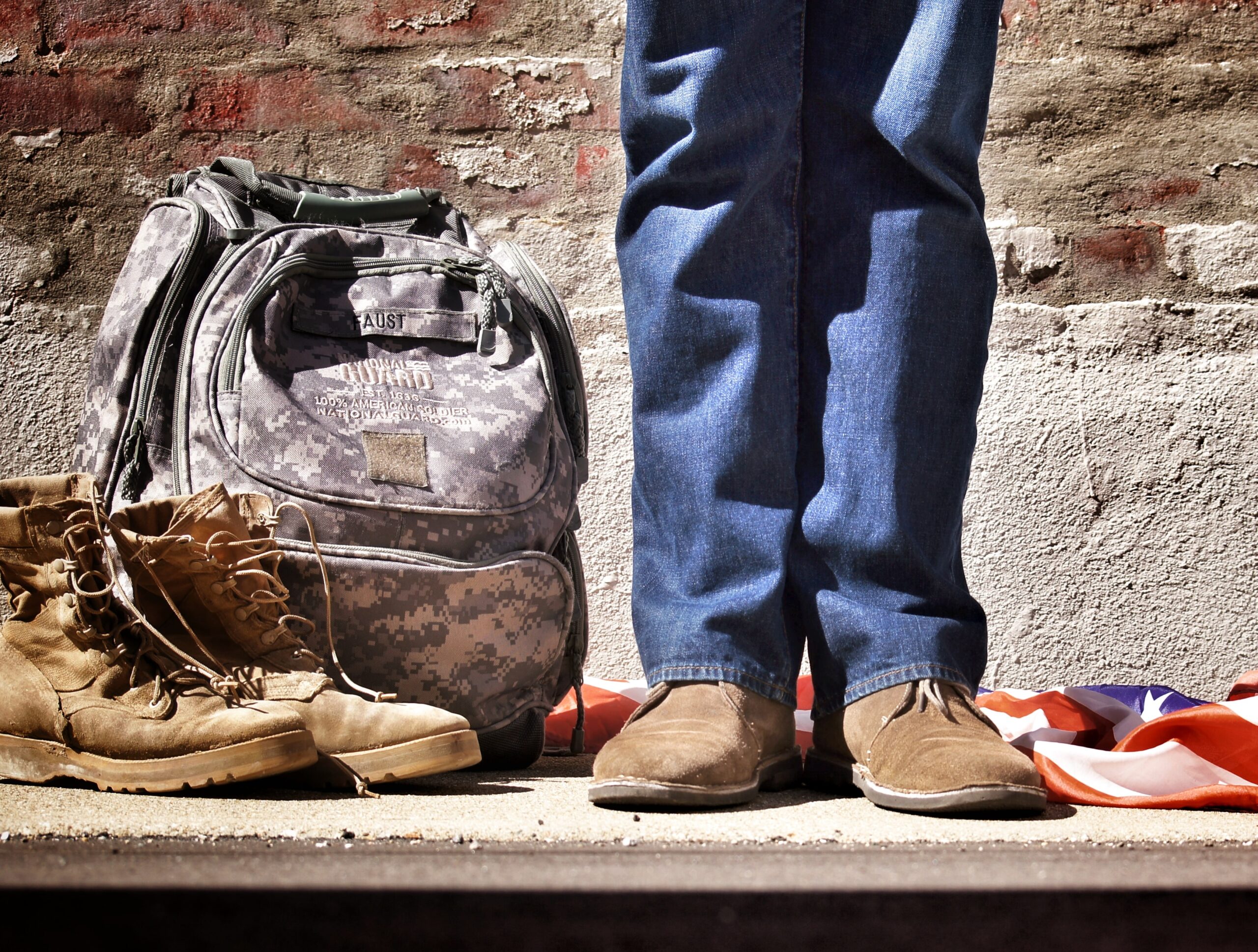
Goals
Our goal is to assess the ability to make accurate predictions of an individual’s significant increase in PTSD symptoms and problematic cannabis use at 1-, 2-, or 3-months using passive data only, and to determine which features contribute to the prediction (e.g., based on one week of passively collected data we can predict significant increase in PTSD at the one month follow-up). We will assess how daily and weekly aggregated self-reported metrics of cannabis use and PTSD symptoms can be used as additional variables to augment the predictive accuracy of our models.
Methods
This project will recruit veterans who screen positively for PTSD and CUD, following discharge from deployment (within 6 months). We will utilize machine learning to improve the ability to detect individuals at risk for escalating PTSD and CUD symptoms.
Data will be collected twice daily for 3 months (i.e., ecological momentary assessment), as well as monthly assessments. Data will include self-reported daily data collection and passive data collected from participants’ phones and wearable devices (e.g., physical activity).
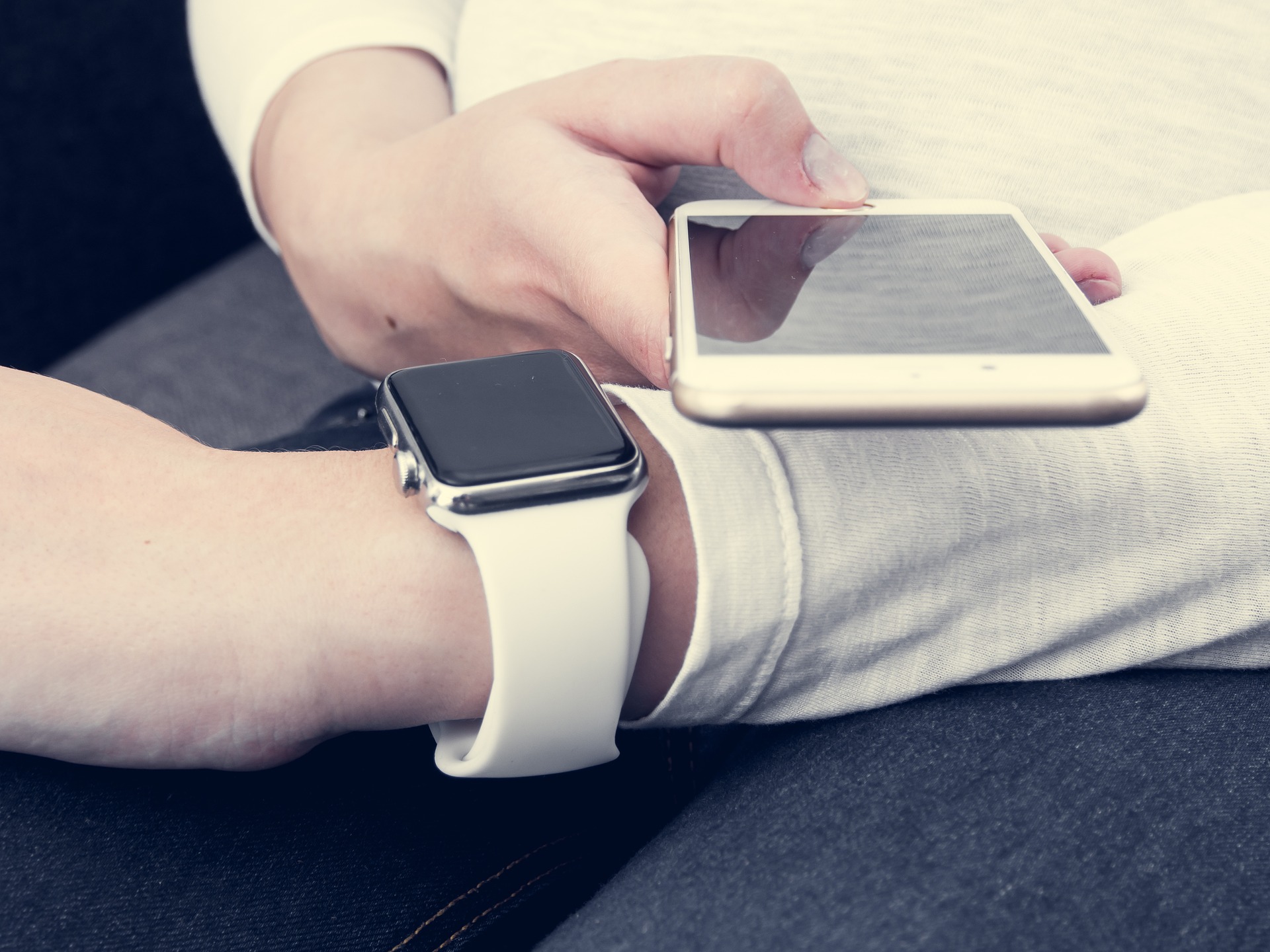
Jordan P. Davis (PI)
Bistra Dilkina (Co-I)
Eric Pedersen (Co-I)
Emily Dworkin (Co-I)
Daniel Leightley (Co-I)
Sriram Nuthi
Praneeth Thota
“Social work and machine learning study aims to identify individualized PTSD interventions for veterans,” USC Suzanne Dworak-Peck School of Social Work, March 11, 2022. https://dworakpeck.usc.edu/news/social-work-and-machine-learning-study-aims-to-identify-individualized-ptsd-interventions-5