Using machine learning for early identification of suicidal ideation among active duty military members
How network dynamics affect a person’s mental health can often be seen most acutely during times of stress and transition. Major military transitions include joining and leaving the military, moving to a different duty assignment in the United States and abroad, and preparing for and returning from deployments. It has been hypothesized that military transitions are inherently stressful because transitions produce significant disruptions in the service member’s social network (1). Service members returning from a combat deployment face many challenges in transitioning to their home environment.
Unfortunately, suicide rates are high among active duty military members. In fact, in 2016, the suicide rate among active duty service members was 21.1 per 100,000 persons, with the highest rate among Army at 26.7 per 100,000 persons (2). By comparison, the suicide rate among the general population in the U.S. in 2017 was 14.1 per 100,000 persons (3).
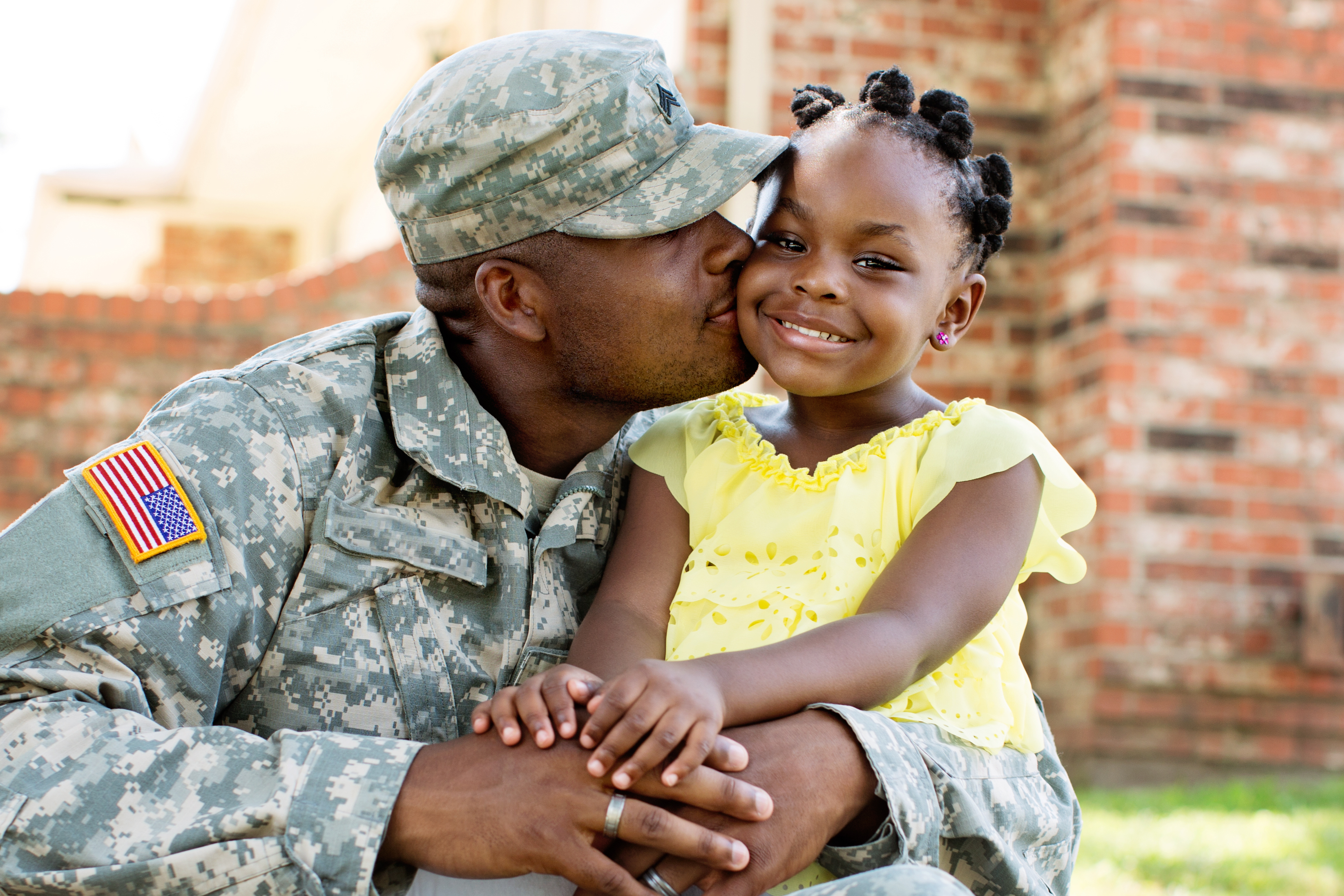
Goals
Although a wealth of research across populations demonstrates the associations between social support and mental health, more sophisticated theorizing and modeling is needed to understand how the networks that surround individuals and the messages transmitted across those network ties interact over time to engender mental states and mental health.
Artificial intelligence (AI) techniques present an opportunity to dynamically model social networks and the messages transmitted across those networks to create predictive models of influence unavailable with standard statistical techniques. Such models hold the promise not only of describing how social networks influence mental health, but also of providing key intervention targets that can inform future programs that act upon social networks.
Methods
We will survey members of an active duty military unit prior to their deployment and conduct follow-up surveys upon their return. Questionnaires will assess mental health and social network compositions. We will then employ predictive analytics to indicate onset of mental health issues based on specific network dynamic patterns. In the past, we have used dynamic Bayesian networks, ensemble decision trees, Gaussian mixture models, and other strategies to infer spatiotemporal patterns in modeling human behavior in data from real-world domains. However because of the dynamic nature of changing networks, we will work toward creating novel AI prediction techniques to be able to make better decisions regarding the timing and type of intervention measures to address negative mental states and prevent suicide and depression among active duty military personnel.
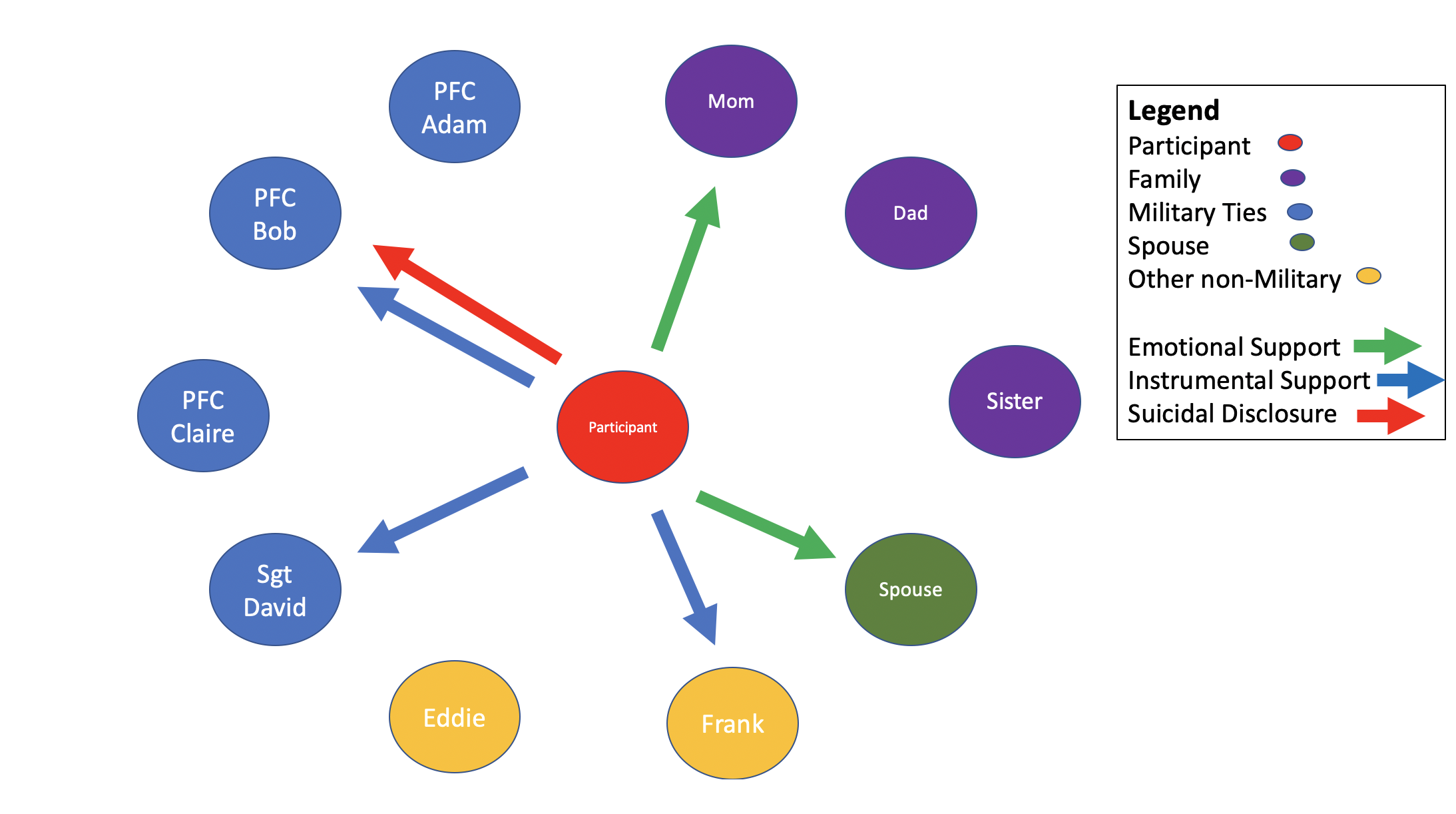
An example of what a soldier’s social network may look like. This does not contain any real data.
If you or someone you know are in crisis, please call the free National Suicide Prevention Lifeline at 1-800-273-TALK (8255) or chat with someone at https://suicidepreventionlifeline.org/chat. Call and chat are available 24 hours a day, 7 days a week. More info here: http://www.suicidepreventionlifeline.org
Aida Rahmattalabi
- EconIntersect, January 15, 2019, Infographic Of The Day: The Growing Problem Of Military Suicides
- Listen.Up.People., September 16, 2018, Podcast Episode 1: Suicide, A public health crisis
- USC Viterbi, May 1, 2018, USC Center for AI in Society Seeks to Reduce Military Suicides
- Castro, C. A., & Kintzle, S. (2014). Suicides in the military: the post-modern combat veteran and the Hemingway effect. Current Psychiatry Reports, 16(8), 460.
- Pruitt, L. D., Smolenski, D. J., Bush, N. E., Skopp, N. A., Edwards-Stewart, A., & Hoyt, T. V. (2017). Department of Defense Suicide Event Report Calendar Year 2016 Annual Report. Retrieved from https://www.pdhealth.mil/sites/default/files/images/docs/DoDSER_CY_2016_Annual_Report_For_Public_Release_508_2.pdf
- American Foundation for Suicide Prevention. (2019). Suicide Statistics. Retrieved from https://afsp.org/about-suicide/suicide-statistics/